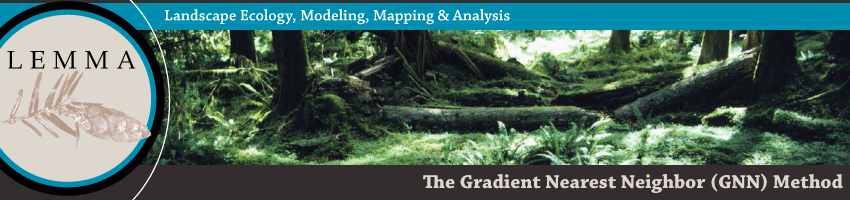
Nearest Neighbor (NN) imputation methods have proven to be an effective tool for characterizing vegetation structure and species composition in forested landscapes across large regions. NN models are particularly well-suited for creating detailed vegetation maps for a variety of reasons: they produce spatially-explicit maps over large areas spanning all ownerships and land uses, they describe multiple attributes of composition and structure, and they maintain covariance among vegetation components (when k=1), and the maps retain the range of variability present in the reference data used to develop the map.
All NN predictions are based on relations between ground (response) data and mapped (explanatory) data. Many variations of NN imputation are possible by varying the distance metric (which quantifies the relations between response and explanatory data), the type of environmental variables used as predictors (e.g. climate, topography) and the number of neighbors (k) imputed to each cell.
Gradient Nearest Neighbor (GNN) is just one variation of NN that the LEMMA group has implemented at broad (regional) spatial extents using regional inventory plots and Landsat imagery, based on k=1 and direct gradient analysis as the 'distance' metric.
The detail-rich nature of GNN models allows users to map the distribution of a wide variety of vegetative characteristics across the landscape. The GNN models are also used as input for several other types of models, including habitat suitability, fuels and fire risk, biomass and carbon, and landscape scenario models for evaluating alternative futures.