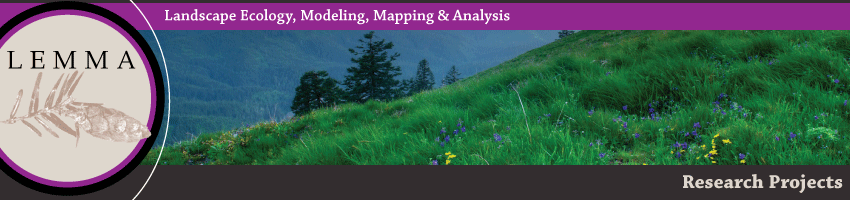
The goal of the Nationwide Forest Imputation System (NaFIS) project, completed in 2009, was to evaluate methods that could be used to produce nationwide data products using nearest neighbor methods. Primary needs for national maps are to provide spatially explicit and statistically valid large- and small-area estimates of forest attributes measured by the Forest Inventory and Analysis (FIA) program of the USDA Forest Service, and to provide detailed data needed for national risk mapping of multiple forest threats. The project used forest inventory data, satellite imagery and ancillary data, and nearest neighbor techniques to construct moderate- (30-m-) resolution datasets of forest attributes to satisfy three strategic objectives:
The NaFIS maps, which are based on 2000 Landsat imagery are served through the NaFIS project website.
The project was separated into eastern and western US components, with the LEMMA group responsible for the study areas in the western US (USGS mapzones 7 [Oregon], 19 [Montana/Idaho], and 28 [Colorado]) and researchers at Michigan State University responsible for the eastern US (USGS mapzones 51 [Michigan] and 63 [Pennsylvania/New York]). The following sections are excerpts from the Western Team Final Report and only refer to the western US study areas completed by LEMMA.
We investigated the consequences of a variety of choices in the modeling process on the accuracy of the resulting maps. We studied issues of scale in summarizing reference plot data. We compared four distance metric choices (referred to as model types): Euclidean (EUC), most similar neighbor (MSN), gradient nearest neighbor (GNN), and random forest nearest neighbor (RAN), and five different values of k: 1, 2, 5, 10 and 20 (the number of neighbors integrated to make each model prediction). We studied the effects of changing modeltype and k values on model accuracy in a variety of dimensions, including plot-level accuracy (root mean square difference, and kappa measures), regional-scale accuracy (assessing for areal bias in the mapped model predictions), and plant community-scale accuracy (summarized from multivariate species abundance predictions at the plot level).
Accuracy varied little across the four model types, although RAN was slightly more effective than the other methods for categorical predictions. Predictions were most accurate when data were summarized within the forest portion of each plot, and whole-plot summaries were second. Accuracy varied greatly across values of k. Higher values of k resulted in a slight increase in plot-level accuracy of our core variables, but also led to a variety of problems for predictions of species abundance (e.g. increasing errors of commission for species predictions, and generating maps that contained unrealistic species combinations).
Due to the simplicity of working with whole plots, we recommend this sampling grain for national implementation, at least in the context of the mountainous west forests, where minor location errors in reference plot data can lead to large mismatches with the spatial data. We also conclude that although RAN would provide slightly higher accuracy than the other model types, it is not yet the best choice for national implementation, as the accuracy gains are minor relative to the costs incurred from longer computing times. Choice of k value appeared more critical than choice of modeltype in generating a map that will be appropriate for multiple uses. The errors with respect to plant community composition incurred with increasing k do not outweigh the gains in plot-level accuracy for structural variables. For applications where forest composition is of interest (e.g., insect and disease modeling, forest succession scenario modeling, estimation of range shifts due to climate change), low values for k will be critical, leading to more realistic estimations of species composition and diversity at any given location.
The LEMMA contributions to this project were supported by the Western Wildland Environmental Threat Assessment Center and the Pacific Northwest Research Station, USDA Forest Service. Landsat imagery and other spatial data were provided by the Remote Sensing Applications Center (RSAC), USDA FS. Other collaborators included the Forest Inventory and Analysis (FIA) Program, USDA FS; State and Private Forestry, USDA FS; and Andrew Finley, Michigan State University.